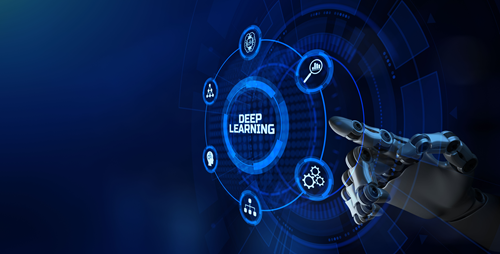
Artificial Intelligence (AI) integration has revolutionized how businesses interact with their customers. The advent of Large Language Models (LLMs) has opened new avenues for creating highly personalized shopping experiences. These sophisticated AI models, capable of understanding and generating human-like text, are now at the forefront of transforming retail into a more customer-centric industry.
By leveraging the power of LLMs, retailers can analyze vast amounts of customer data, including past purchases, browsing history, and preferences, to offer highly tailored recommendations and services. This level of personalization enhances the shopping experience and fosters a deeper connection between the brand and its customers. As a result, LLMs are becoming an indispensable tool in the arsenal of retailers who aim to stay competitive and relevant in a market where personalization is no longer a luxury but a necessity.
The Emergence of Personalized Shopping Experiences
The concept of personalized shopping isn’t new, but how AI, specifically LLMs, is reshaping it is groundbreaking. By analyzing customer data, preferences, and past shopping behaviors, LLMs can generate tailored product recommendations personalized marketing messages, and even offer individualized customer support. This level of customization was once a distant dream but is now a tangible reality due to AI and deep learning advancements.
Training LLMs for Retail: The DeepSpeed Advantage
Training LLM for AI to cater to the specific needs of the retail sector is a complex task. It requires processing vast amounts of data to accurately understand customer preferences and shopping patterns. This is where DeepSpeed training comes into play. DeepSpeed, an optimization library for deep learning, significantly enhances the efficiency of training LLMs. By optimizing memory usage and accelerating computation, DeepSpeed enables the training of larger, more sophisticated models that can deliver more accurate and nuanced insights into customer behavior.
DeepSpeed’s Zero Optimization Stage in LLM Training
One of the critical aspects of training LLMs with DeepSpeed is selecting the appropriate ZeRO (Zero Redundancy Optimizer) optimization stage. When struggling with the question, what should be your DeepSpeed’s Zero optimization stage?— the choice of ZeRO stage – whether it’s ZeRO-1, ZeRO-2, or ZeRO-3 – depends on the specific requirements of the training process. For instance, in a retail context where the model needs to process extensive customer data sets, a higher ZeRO stage might be more beneficial. This decision is crucial in ensuring that the LLMs are powerful and efficient in handling the vast data inherent in the retail industry.
The Impact of LLMs on Customer Engagement and Sales
Let’s explore the impact of Large Language Models (LLMs) on customer engagement and sales in the retail sector:
- Enhanced Customer Insights: LLMs can analyze vast amounts of customer data through their advanced natural language processing capabilities. This analysis includes studying past purchase history, browsing patterns, and social media interactions. By doing so, these models gain deep insights into individual customer preferences and behaviors.
- Tailored Product Recommendations: LLMs can curate highly personalized product recommendations with the insights gained. Unlike generic recommendations, these are tailored to each customer’s unique preferences, making them more relevant and appealing. This relevance significantly increases the likelihood of purchase.
- Improved Customer Interaction: LLMs can power chatbots and virtual assistants to provide real-time, personalized customer service. These AI-driven interactions are efficient and mimic human-like understanding, making customers feel more valued and understood.
- Predictive Analytics for Demand Forecasting: LLMs can predict future buying trends based on current data trends. This predictive capability allows retailers to stock products more aligned with anticipated customer demands, reducing overstock and understock scenarios.
- Dynamic Pricing Strategies: By understanding customer price sensitivity and market trends, LLMs can assist in developing active pricing strategies. This approach ensures that prices are optimized for sales conversion and profit margins.
- Increased Customer Retention: Personalized experiences foster customer loyalty. Customers are more likely to return to a retailer that consistently understands and meets their needs effectively, thereby enhancing long-term customer retention.
- Data-Driven Marketing Campaigns: LLMs enable retailers to create more targeted and effective marketing campaigns. By understanding customer segments more deeply, these campaigns can be tailored to resonate with the intended audience, leading to higher engagement rates.
The Future of Retail with AI and LLMs
Looking ahead, the role of AI and LLMs in retail is set to grow even more. As these technologies evolve, they offer even more sophisticated and nuanced ways to enhance the shopping experience. From virtual shopping assistants to AI-driven inventory management, the possibilities are endless. Retailers who embrace these technologies will be well-positioned to stay ahead in the competitive market.
Conclusion: Optimizing LLMs for the Future of Retail
In conclusion, optimizing LLMs, mainly through tools like DeepSpeed, is crucial in harnessing their full potential in the retail sector. As we move forward, LLM optimization will remain a key focus area, ensuring that these models are powerful but efficient and effective in delivering personalized shopping experiences. The future of retail lies in the hands of AI, and LLMs are leading this transformative journey.

Tech Hub Digital, a one-stop destination for complete technology-related information.